The Unresolved Tension Between AI and Learning
If education is accelerated using AI, will we lose some crucial aspects of learning that will prove to be problematic?
by Dan Cohen
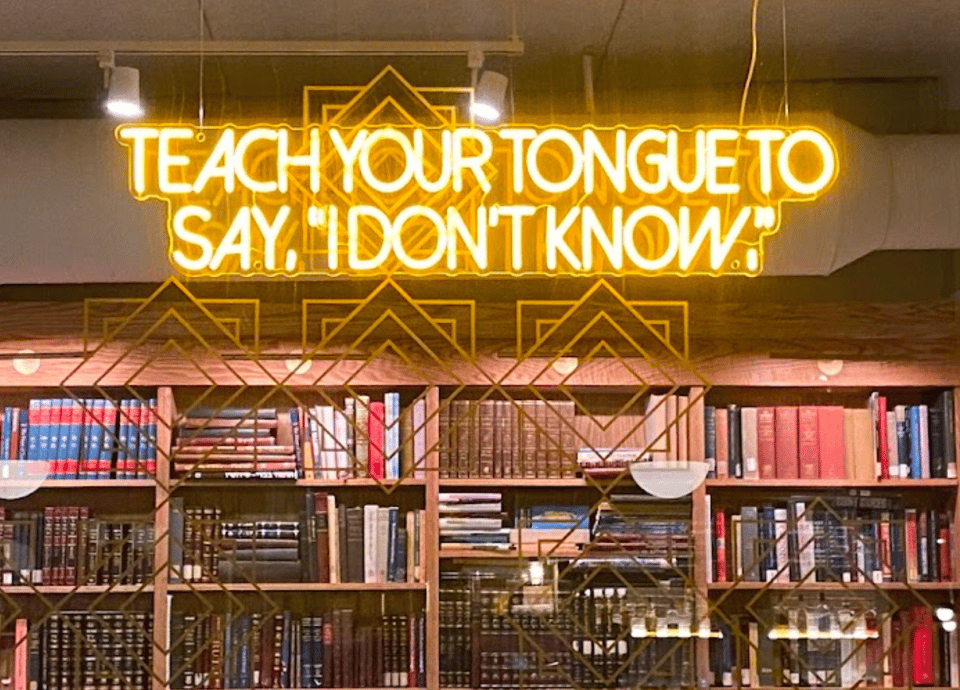
The act of learning is a complicated and somewhat mysterious process, but more or less it is a measured progression, one that takes time. We read books or articles on a topic, or listen to an instructor and interact with fellow students, or have other experiences that all relate to a common subject. Each of these moments settles like sediment in the ocean of our mind, and are compacted under their accumulating weight until they form the solid foundation of understanding. Then we are on firmer ground when we learn more in this area of knowledge, and have the basis to erect new concepts and creations.
Learning is demanding because our brains require numerous prompts and constant reinforcement to internalize information. Even if we are decades beyond the heyday of rote memorization and classroom repetition, and into more qualitative, experiential, and collaborative pedagogical modes, learning is still a process that takes time, and that is often difficult. When we begin to learn something, we are slow, deliberative, and clumsy; at the end, we are swift, intuitive, and facile.
It is a silly Hollywood montage, but the classic “wax on/wax off” scene in The Karate Kid, in which the titular kid’s prowess emerges from the tedium of buffing a car day after day for Mr. Miyagi, does reflect a basic truth. Some tasks along a learning journey may seem menial — reviewing vocabulary, practicing scales, or looking at thousands of freckles — but these activities eventually allow one to speak a foreign language, solo on guitar, or assess a skin blemish in seconds if you’re a dermatologist. Process over time leads to expertise. That process often has to be mentally taxing, involving masses of information, variance, and the acquisition of tacit knowledge as well as knowledge we can articulate.
What happens, then, when a new technology purports to vastly accelerate learning as AI is supposed to do? What if learning became effortless, guided by an AI tutor far superior to any human? If we no longer need a lengthy process of granular accumulation that ends in a mountain of expertise, will we have truly learned? If a robot arm does some of the waxing, will we be able to punch in the ring without it?
Some early studies should give us pause. In “Generative AI Can Harm Learning,” Hamsa Bastani, Osbert Bastani, Alp Sungu, Haosen Ge, Ozge Kabakcı, and Rei Mariman took a large sample of high school students in a math class, and gave one half access to a clone of ChatGPT-4, which could assist the student with every step of solving a problem set. They gave another cohort a more circumscribed version of a GPT that disallowed some generative AI features. The limited model could “provide hints to the student without directly giving them the answer.” This is what happened:
Consistent with prior work, our results show that access to GPT-4 significantly improves performance…However, we additionally find that when access is subsequently taken away, students actually perform worse than those who never had access…Our results suggest that students attempt to use GPT-4 as a “crutch” during practice problem sessions, and when successful, perform worse on their own. Thus, to maintain long-term productivity, we must be cautious when deploying generative AI to ensure humans continue to learn critical skills.
Those in the first cohort, who were allowed to use AI as most students are using these tools right now, improved greatly on their math assessments over the duration of the study (up 50%!), but when they were asked to do a math test without AI help later on, they scored worse (down 17%!). This makes sense given our understanding of true learning. AI accelerated the students’ ability to solve math problems, but didn’t allow them to acquire an innate understanding. They didn’t get a feel for mathematics, a sense of how it actually works; they were merely able to do assignments faster and more accurately.
The second cohort, which could only ask their AI for advice, clarifications, or parallels from other problems, had to sweat it out more to get to the answers. This friction was fruitful, and it showed in their retention and longer-term mathematical ability. Guardrails around the AI led to much improved outcomes.
So the key question educators face right now is this: Are we using AI to enhance learning, or to replace some learning steps that turn out to be essential? And what are students doing on their own, out of the view of instructors? Here in academia, virtually every university has set up courses for faculty on how to integrate AI effectively in the classroom. Many of those courses implicitly agree with the conclusions of Bastini et al.’s paper, that you can’t just let students loose with raw AI, that you have to shape their use to supplement learning rather than detract from it. But let’s face it — students spend most of their time outside the classroom. Is there any way to mandate that they use tailored or circumscribed AI, forcing them to do it the complex and hard way rather than the simple and easy way, to ensure deep learning?
Some might argue that, as with the addition of the calculator in education decades ago, in the real world of work, students will continue to have access to new technology. They will be using maximal, unguardrailed AI in their careers, so they might as well learn a discipline in the way it will be practiced in the future. But guess what is happening right now: Employers are already realizing that a relative novice armed with AI, although perhaps competent enough to get work done, is far less valuable than an experienced practitioner who has a sturdy mental model of a domain.
In “Artificial Intelligence, Scientific Discovery, and Product Innovation,” Aidan Toner-Rodgers looked at a commercial research laboratory in material science in which the researchers were given access to a specialized AI tool. As with the high school students, these professionals generally did their work faster and better with AI as their collaborator, but there was a marked difference in effectiveness between the early career scientists and the senior ones. Those who had done years of lab work, who had read countless articles in the field, who had a traditional education long before AI, were far more facile in improving their work when given the AI tool. Their deep foundational knowledge allowed them to intuit the most promising candidates for new materials as the bot generated possibilities. Those who had to supplement more of their brain with the AI — the younger researchers — saw much more limited gains, and had trouble discerning good AI outputs from poor ones. (The study also showed, rather depressingly, that “82% of [the] scientists report reduced satisfaction with their work due to decreased creativity and skill underutilization” after they were given the AI tool.)
So it seems likely that those who will benefit from AI the most are those who have already done the hard labor of traditional, non-AI learning, who already have the bedrock foundation of knowledge and intuition. Toner-Rogers’s study thus reinforces the point I made last year in “Is Science Becoming Conceptual Art?” The combination of the AI autocompletion of scientific processes and lab automation holds the potential to greatly shorten the distance between a scientific hypothesis and experimental confirmation. In this wonderful world of accelerated science, however, the middle steps formerly tackled by early stage scientists — tomorrow’s future conjurers of breakthroughs — are erased. This, paradoxically, leads to a chasm in the profession in which there is no experiential on-ramp to the fast lane of the principal investigator, no training that gives you the rich base of knowledge and intuition needed to develop your field further.
“My house is on the median strip of a highway,” the absurdist comedian Stephen Wright once said. “You don't really notice, except I have to leave the driveway doing 60.” The risk right now is that without a solid educational theory — and practice — of integrating AI into learning, we will be asking our students to leave college doing 60, and their engines won’t have the octane to handle that kind of acceleration.