Books are Big AI's Achilles' Heel
AI companies may have the money and the data centers, but they are badly in need of what humble libraries have in abundance
by Dan Cohen
I recently joined the board of Authors Alliance, a nonprofit that seeks to “advance the interests of authors who want to serve the public good by sharing their creations broadly.” This week Authors Alliance celebrates its 10th anniversary, and as part of the celebration the organization has been posting pieces on urgent issues related to writing and technology. My contribution, co-written with Authors Alliance’s executive director, Dave Hansen, is reposted here: “Books are Big AI's Achilles Heel.”
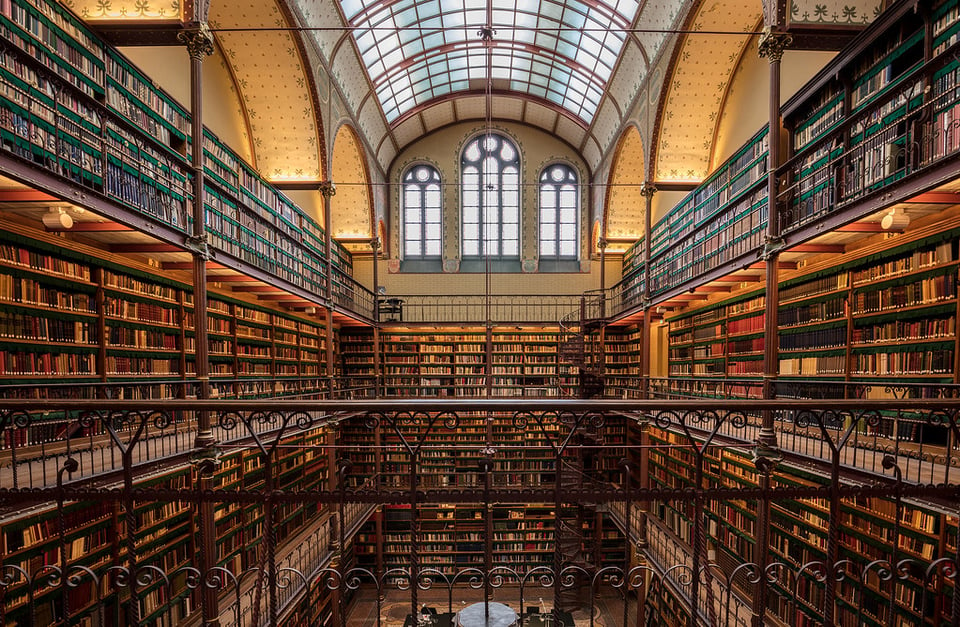
Rapidly advancing artificial intelligence is remaking how we work and live, a revolution that will affect us all. While AI’s impact continues to expand, the operation and benefits of the technology are increasingly concentrated in a small number of gigantic corporations, including OpenAI, Google, Meta, Amazon, and Microsoft.
Challenging this emerging AI oligopoly seems daunting. The latest AI models now cost billions of dollars, beyond the budgets of startups and even elite research universities, which have often generated the new ideas and innovations that advance the state of the art.
But universities have a secret weapon that might level the AI playing field: their libraries. Computing power may be one important part of AI, but the other key ingredient is training data. Immense scale is essential for this data—but so is its quality.
Given their voracious appetite for text to feed their large language models, leading AI companies have taken all the words they can find, including from online forums, YouTube subtitles, and Google Docs. This is not exactly “the best that has been thought and said,” to use Matthew Arnold’s pointed phrase. In Big AI’s haphazard quest for quantity, quality has taken a back seat. The frequency of “hallucinations”—inaccuracies currently endemic to AI outputs—are cause for even greater concern.
The obvious way to rectify this lack of quality and tenuous relationship to the truth is by ingesting books. Since the advent of the printing press, authors have published well over 100 million books. These volumes, preserved for generations on the shelves of libraries, are perhaps the most sophisticated reflection of human thinking from the beginning of recorded history, holding within them some of our greatest (and worst) ideas. On average, they have exceptional editorial quality compared to other texts, capture a breadth and diversity of content, a vivid mix of styles, and use long-form narrative to communicate nuanced arguments and concepts.
The major AI vendors have sought to tap into this wellspring of human intelligence to power the artificial, although often through questionable methods. Some companies have turned to an infamous set of thousands of books, apparently retrieved from pirate websites without permission, called “Books3.” They have also sought licenses directly from publishers, using their massive budgets to buy what they cannot scavenge. Meta even considered purchasing one of the largest publishers in the world, Simon & Schuster.
As the bedrock of our shared culture, and as the possible foundation for better artificial intelligence, books are too important to flow through these compromised or expensive channels. What if there were a library-managed collection made available to a wide array of AI researchers, including at colleges and universities, nonprofit research institutions, and small companies as well as large ones?
Such vast collections of digitized books exist right now. Google, by pouring millions of dollars into its long-running book scanning project, has access to over 40 million books, a valuable asset they undoubtedly would like to keep exclusive. Fortunately, those digitized books are also held by Google’s partner libraries. Research libraries and other nonprofits have additional stockpiles of digitized books from their own scanning operations, derived from books in their own collections. Together, they represent a formidable aggregation of texts.
A library-led training data set of books would diversify and strengthen the development of AI. Digitized research libraries are more than large enough, and of substantially higher quality, to offer a compelling alternative to existing scattershot data sets. These institutions and initiatives have already worked through many of the most challenging copyright issues, at least for how fair use applies to nonprofit research uses such as computational analysis. Whether fair use also applies to commercial AI, or models built from iffy sources like Books3, remains to be seen.
Library-held digital texts come from lawfully acquired books—an investment of billions of dollars, it should be noted, just like those big data centers—and libraries are innately respectful of the interests of authors and rightsholders by accounting for concerns about consent, credit, and compensation. Furthermore, they have a public-interest disposition that can take into account the particular social and ethical challenges of AI development. A library consortium could distinguish between the different needs and responsibilities of academic researchers, small market entrants, and large commercial actors.
If we don’t look to libraries to guide the training of AI on the profound content of books, we will see a reinforcement of the same oligopolies that rule today’s tech sector. Only the largest, most well-resourced companies will acquire these valuable texts, driving further concentration in the industry. Others will be prevented from creating imaginative new forms of AI based on the best that has been thought and said. As they have always done, by democratizing access libraries can support learning and research for all, ensuring that AI becomes the product of the many rather than the few.
Further reading on this topic: “Towards a Books Data Commons for AI Training,” by Paul Keller, Betsy Masiello, Derek Slater, and Alek Tarkowski.