Break Expectations
Where does the ability of AI to mimic human expression end? Poetry provides a helpful case study
by Dan Cohen
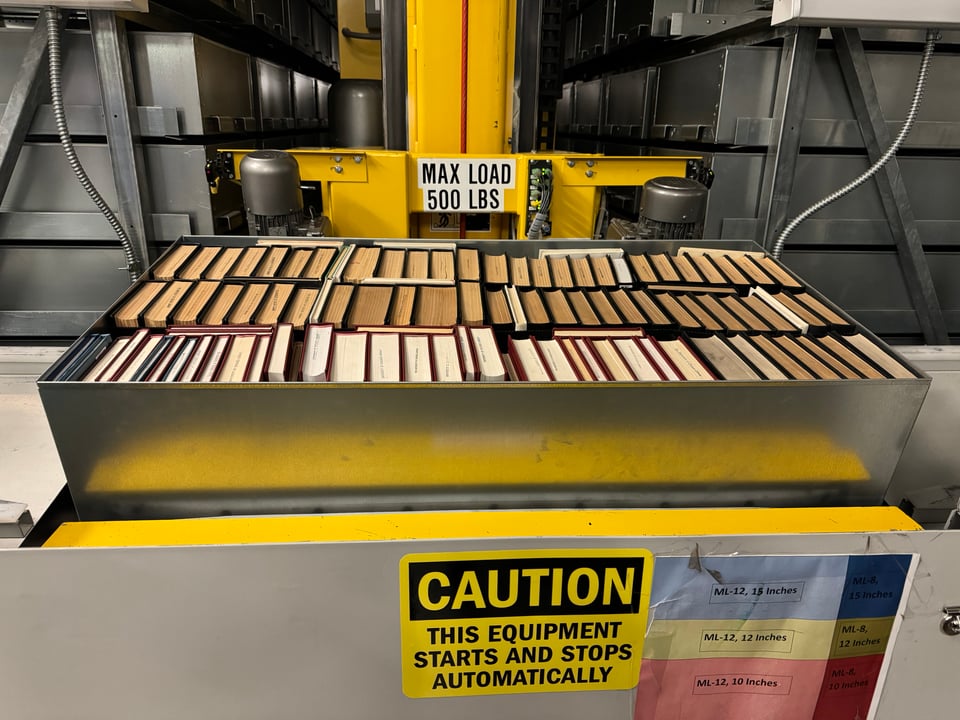
In his analysis of the novel I covered in the last issue of this newsletter, Robin Sloan’s Moonbound, Alan Jacobs concludes:
We love the old stories — we love stories that do what we expect them to do, what we know in advance they will do. But we also love it when they surprise us. Repeatedly in Moonbound we are told that “the great question of the Anth” [i.e., humans] is: “What happens next?” And we only ask that question when a story is surprising us, or when we hope it will.
We need themes, and we need variations on themes.
Alan and I both use the shorthand “off-script” for these crucial deviations from the norm. The tension between expectations and the element of surprise, the script and the off-script, seems like an especially essential topic right now. Machine learning and generative AI, by processing large collections of text and images, have greatly strengthened the science of pattern recognition and, in turn, the automation of pattern duplication, thus potentially weakening our understanding of how and why patterns break and expectations are dashed.
Alan highlights the importance of luck in the zigzag; in this space over the last two years I have tried to understand the role of human agency and individuality. Both are mysterious entities that confound predictability and divert the linear timelines of narratives and history. (Previously in Humane Ingenuity: the inflections of opera singers; no shortcuts to a personal and effective compositional style; how idiosyncratic reading leads to unpredictable writing, and the relationship between lived experience and great art.)
In this context, studying the boundaries between common forms and unexpected variance in human expression is of paramount importance. Take poetry, one of our most treasured and complicated modes of writing. In a new study, “Sonnet or Not, Bot? Poetry Evaluation for Large Models and Datasets,” Melanie Walsh, Anna Preus, and Maria Antoniak ask the latest AI models to categorize thousands of poems.
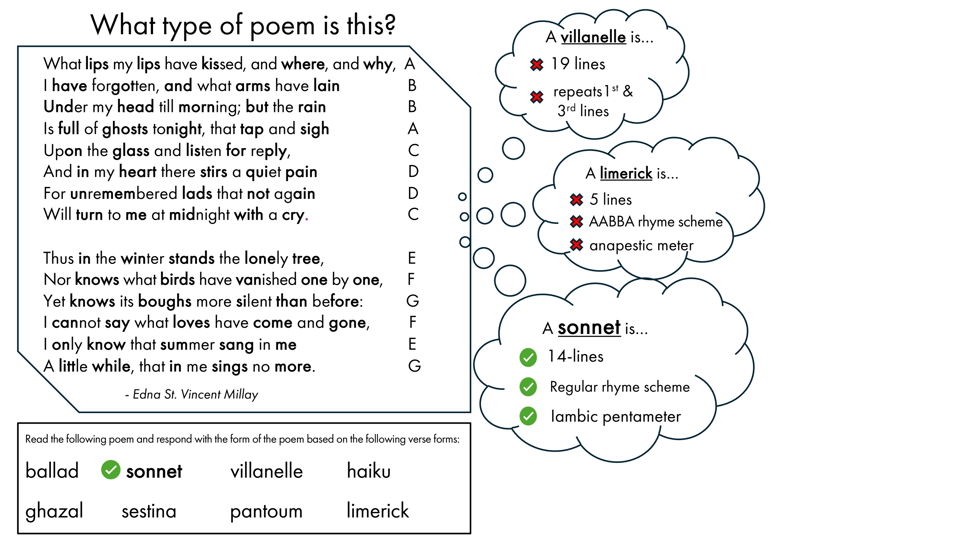
The models are impressively able to discern a sonnet from a villanelle, often with greater accuracy than the human panel the authors have assembled — within certain bounds.
We find that LLMs — particularly GPT-4 and GPT-4o — can successfully identify both common and uncommon fixed poetic forms, such as sonnets, sestinas, and pantoums, at surprisingly high accuracy levels when compared to annotations by human experts.
But identifying haikus or limericks, which have rigid anatomies, is much easier than grasping the flexible musculature of a sophisticated poem. Good poetry always aims to transcend mere forms, as the authors of the article beautifully describe:
Poetic features uniquely combine verbal, aural, and visual elements; the substance, sound, and (in written poetry) appearance of words on the page (e.g., white space) all matter. What’s more, poetry often communicates deep emotion and meaning in non-literal, ambiguous ways, employing figurative language, irony, and allusion.
This vitality that floats above the stanzas is the reason that the accuracy of the LLMs declines significantly as they are asked to describe more fluid — and more surprising — elements of distinctive poems.
Performance varies widely by poetic form and feature; the models struggle to identify unfixed poetic forms, especially ones based on topic or visual features.
For “fixed” forms, there are often specific rules and complex patterns of versification, but these rules are also likely to be stretched or broken by poets.
Walsh, Preus, and Antoniak usefully adopt Peter Seitel’s theory of genres, summarized in the crisp phrase that genres are “frameworks of expectation, established ways of creating and understanding that facilitate human interaction and the communication of meaning” (an idea Seitel borrows, in part, from William F. Hanks). The way that stories conform to certain sequences (e.g., in the final act of a mystery, the murderer will be revealed) greatly enhance the broad acceptability and power of those stories. A genre, Seitel explains, “articulates principal themes in logically expected places to maximize the effect and efficiency of performance.”
The best poets, the best writers, the best artists, and the best musicians break these expectations, however. Genres help to convey themes through common, recognizable formats, but in this shared human system of communication, subverting those very themes, disordering the orderly, becomes a superpower. We need themes, and we need variations on themes. Currently — eternally? — AI is not very good at the latter, either in identifying potent deviation or in generating it.
The big AI vendors have tried their best to cloud the difference between replicant poetry and human poetry, with chatbots named “Bard” and models named “Sonnet” and “Haiku,” as the authors of “Sonnet or Not” cheekily note. It has been effective marketing; just this week there was an article in the New York Times with the headline “AI Can Write Poetry, but It Struggles With Math.”
Well, yes, AI can create poetry, if by that you mean verses that dutifully conform to frameworks of expectation. But how can it break those expectations in meaningful ways and thus convey something more genuine to its audience? In the reference website for Copilot, Microsoft trumpets their AI’s ability to write poetry, from an acrostic to a quatrain. Heck, why not use it, they suggest, to automate the writing of a poem to your loved one?
To which the only truly human reaction can be profound shame
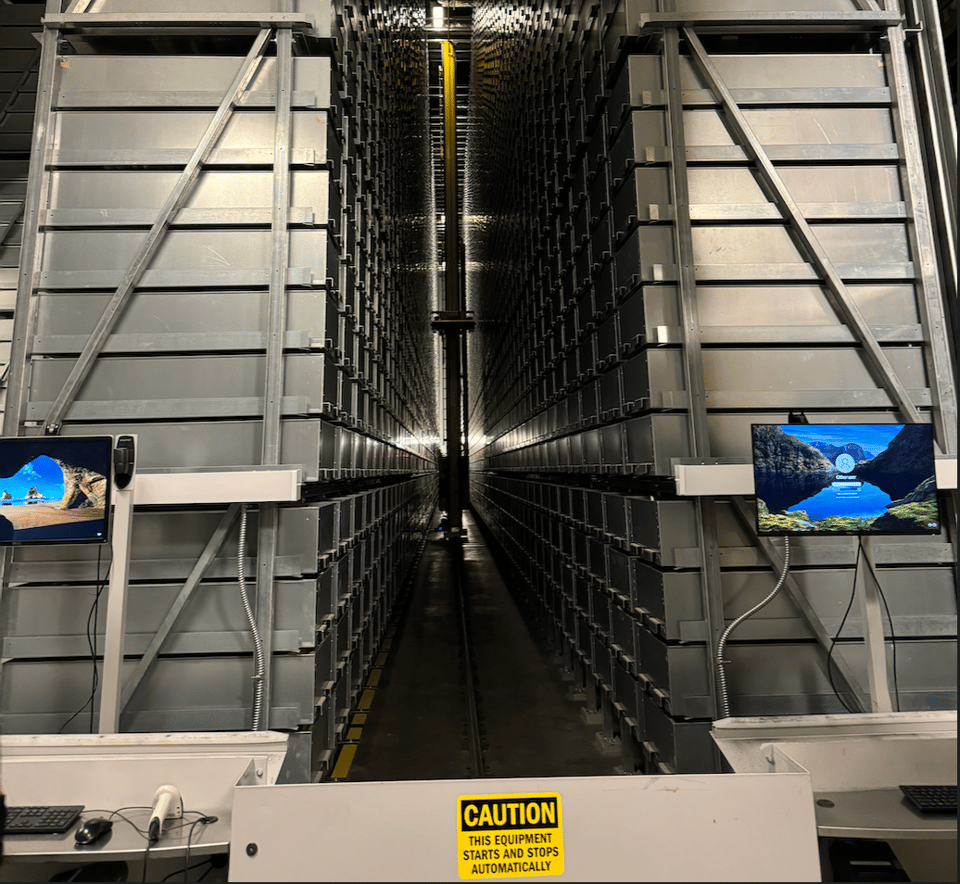